Text analytics: Everything you need to know to create real customer experience impact
Published on Mar 17, 2020
With rapid investment in customer experience management (CEM) platforms, leading brands know the quickest path to growth starts with listening to customers. But an increasingly complex customer journey spanning multiple touchpoints—many with distinct feedback channels—can make surfacing actionable insights seem like a daunting task.
To drive real change, brands require enterprise-level platforms that translate mountains of data into targeted insights that drive business outcomes—consistently and efficiently. And with customers growing weary of lengthy surveys asking about every aspect of their experience, open-ended comments offer enormous potential for streamlining the feedback-to-insight process.
But just because brands know it’s important doesn’t mean they’re doing it effectively. Most text analytics technologies either can’t handle the volume and complexity of the data being processed or they’re so convoluted that it’s difficult to know where to start.
How can brands ensure their text analytics solution is providing the level of insight they need? Let’s look at 3 steps you can take to unpack the complexities of text analytics and formulate a strategy that will optimize customers’ open-ended feedback:
1. Demystify buzzwords for a foundational understanding of text analytics
Before you can properly evaluate your current text analytics solution or determine the right tech investment for the future, you need to be familiar with the lingo. Here are some definitions to help you brush up on the technical terms of text analytics:
- Artificial intelligence (AI)—Advanced computer systems that are able to perform tasks normally requiring human intelligence—such as visual perception, speech recognition, decision-making, and translation
- Entity ontology—A set of concepts and categories in a department that shows their properties and the relations between them; in regard to text analytics, entity ontologies are used to tag + categorize the products and services mentioned in customer comments so they align with a brand’s internal product hierarchy
- Machine learning—The scientific study of algorithms and statistical models that computer systems use to effectively perform a specific task without using explicit instructions, relying on patterns and inference instead
- Natural language processing—A subfield of linguistics, computer science, information engineering, and artificial intelligence concerned with the interactions between computers and human languages—in particular how to program computers to process and analyze large amounts of natural language data
- Predictive analytics—The use of data, statistical algorithms, and machine-learning techniques to identify the likelihood of future outcomes based on historical data; the goal is to go beyond knowing what has happened to providing a best assessment of what will happen in the future
- Sentiment analysis—The process of computationally identifying and categorizing opinions expressed in a piece of text, especially in order to determine whether the attitude toward a particular topic, product, etc. is positive, negative, or neutral
- Text analytics—The process of converting unstructured text data into meaningful data for analysis—measuring customer opinions, product reviews, feedback, and sentiment to support fact-based decision-making
These terms can be tricky, but knowing the basics is the first step in implementing a successful text analytics strategy. Once you have a solid foundation, you’ll understand what’s applicable to your CEM program and how to best incorporate the solution.
2. Evaluate the text analytics capabilities that truly matter to the CX industry
Next, it’s time to thoroughly vet providers to find the right fit for your needs. Not every text analytics solution is equal. Some will take a bare-minimum, DIY approach—leaving the heavy lifting to you and your IT team. Others will flaunt fancy features that seem promising, but are wrapped up in a confusing interface. The best text analytics technology will be accurate, powerful, and intuitive by providing:
- Rich input: All open-ended CX feedback—across all touchpoints—should be evaluated. Incorporate comments from location, online, contact center, social, and third-party sources (e.g., Salesforce, Ziosk).
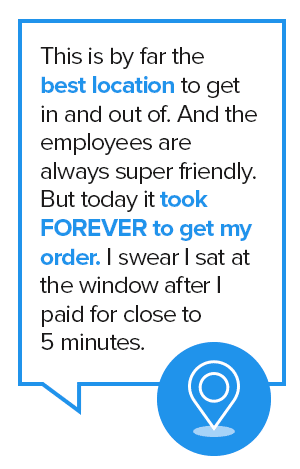
Top-tier accuracy: You need customized libraries built for your specific industry, so the text analytics engine will look for relevant categories and terms. In addition, you shouldn’t have to depend on manual coding. Find a provider using human-assisted machine learning with complex algorithms that continuously fine-tune sentiment accuracy.
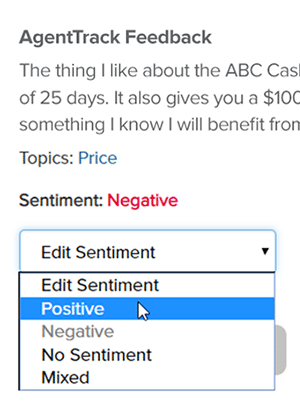
Powerful reporting: You need to be able to visualize your verbatim feedback—seeing aggregated scores across all feedback sources—so you can understand the data holistically. Plus, you need to be able to slice and dice the data so you can translate that omnichannel feedback into actionable insights.
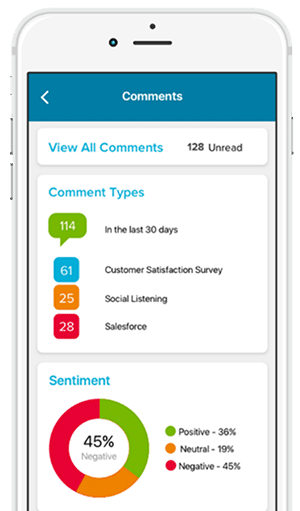
- Next-level insights: When you combine an accessible, easy-to-use, and intuitive platform with hands-on professional services, you’ll get the kind of insights that drive real change. With the support of a dedicated team—one using text analytics alongside other datasets—you can dig deeper to uncover meaningful context around customer issues.
Brands must thoroughly evaluate the functionality of a text analytics platform prior to making investment decisions. Forrester states “as more firms invest in voice-of-the-customer (VoC) technologies, vendors' integration capabilities, text analytics, and service offerings will dictate where the money is best spent… Buyers should look at the industry ontologies a vendor supports, the languages available, and how the text analytics technology works—whether it is rules-based, supervised machine learning, unsupervised machine learning, or a hybrid. Buyers should have vendors do a live proof of capabilities during technology demonstrations— enabling the buyers to get a feel for the tool's true out-of-box functionality."
3. Combine data for a holistic view of the customer experience
And finally, be sure to break down data silos and integrate your data. Just like survey scores don’t exist in a vacuum, neither do comments—and you need to understand the relationship between both to get the full picture. Every iteration of the customer experience offers an opportunity to gain a more nuanced understanding of where to improve.
Brands must bridge quantitative and qualitative data. Correlating mentions and measures will help draw a direct line between what’s being talked about and how those topics affect the experience. A solution that integrates text analytics with other datasets will help brands:
- Understand how product mentions correlate to Overall Satisfaction, loyalty metrics, + KPIs
- Track frequency of mentions to gauge success of new products + services to forecast demand
- Right-size product innovation strategies to ever-evolving customer expectations
Whether it’s specific products, new service standards, or recent operational changes, noting when customers call out those experiential variables—and more importantly, understanding how they impact important measures—is mission critical to building an innovation strategy that resonates.
Unveil the insights hiding in unstructured data
More than ever, brands need robust, intuitive tools designed with end users in mind—especially when it when it comes to input as complicated as the unstructured data found in customer comments. The right text analytics technology will provide the actionable insights you need to drive meaningful change in your business.
For more on how text analytics helps brands mine customer comments for deeper insights, check out this video on text analytics technology.
Related articles
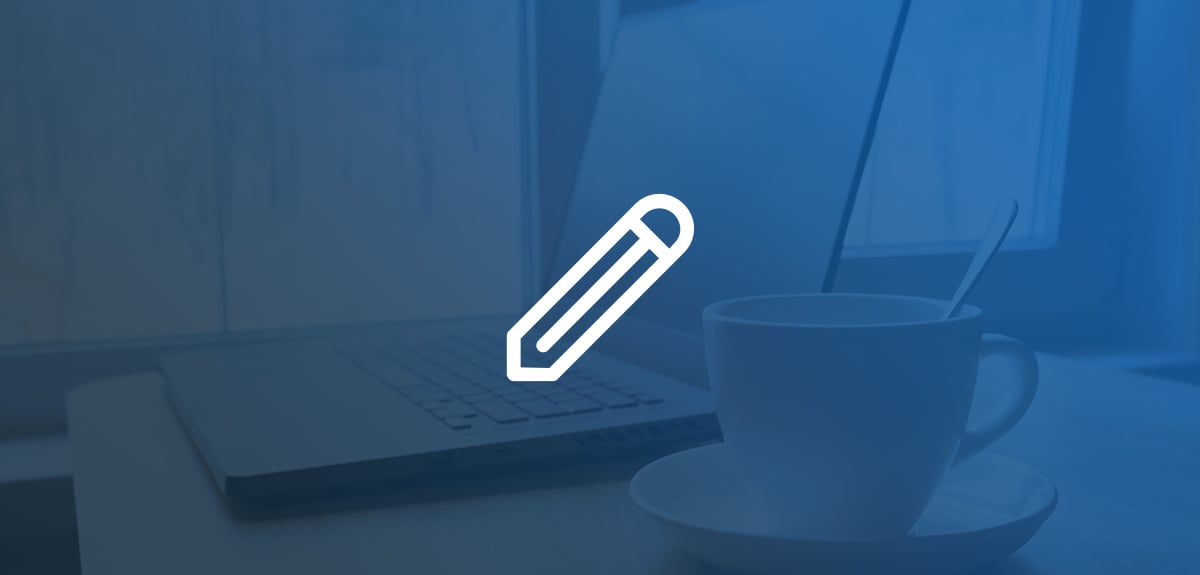
Turn Open-Ended Feedback into Actionable Insights With Text Analytics
Leading brands know the quickest path to growth starts with listening to customers. But an increasingly complex customer journey spanning multiple touchpoints can make surfacing actionable insights se
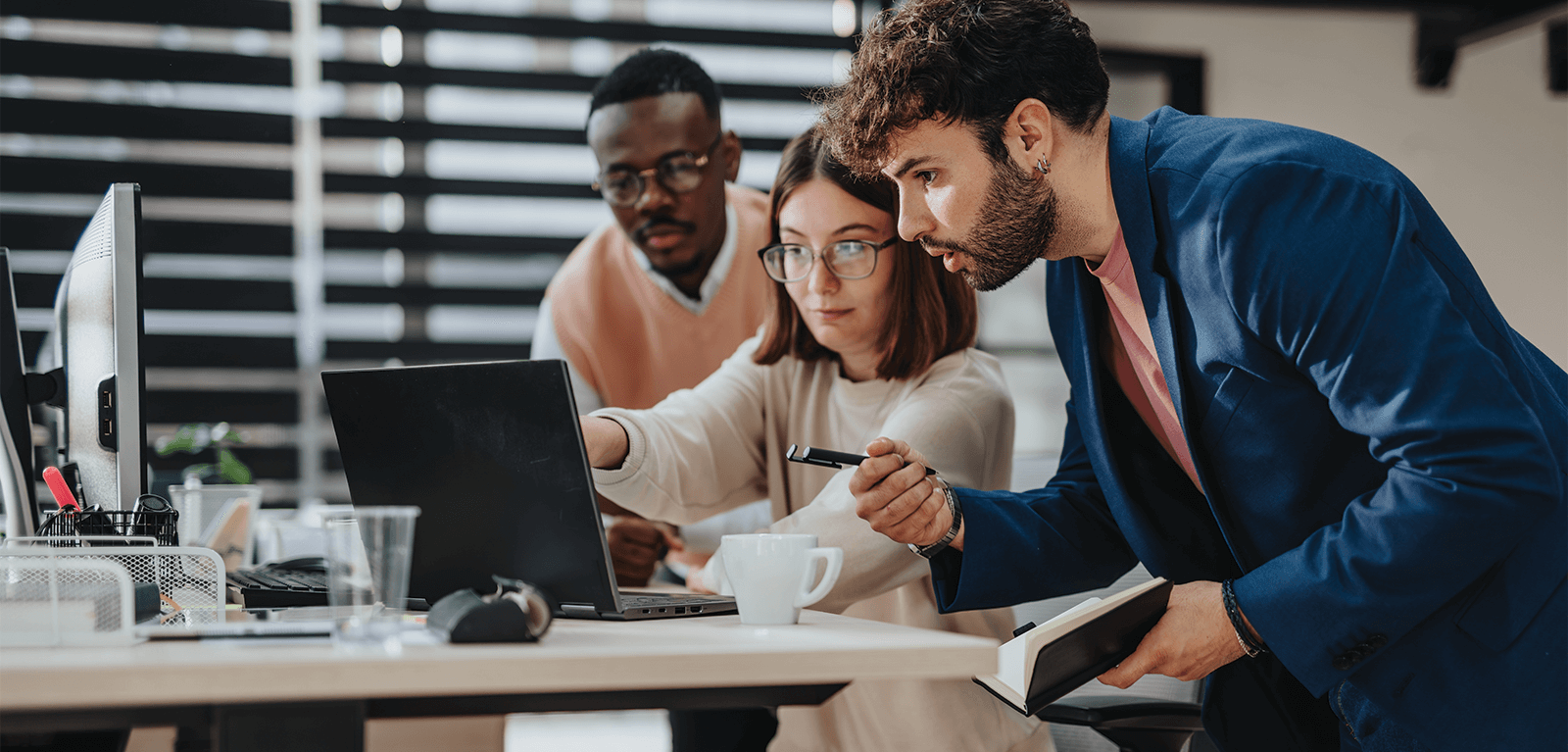
Does your customer experience management software give you insights… or homework?
Turning data into actual insights is a hurdle many in the customer experience game are trying to clear. Your customer experience (CX) software might provide you with a large amount of customer data, b
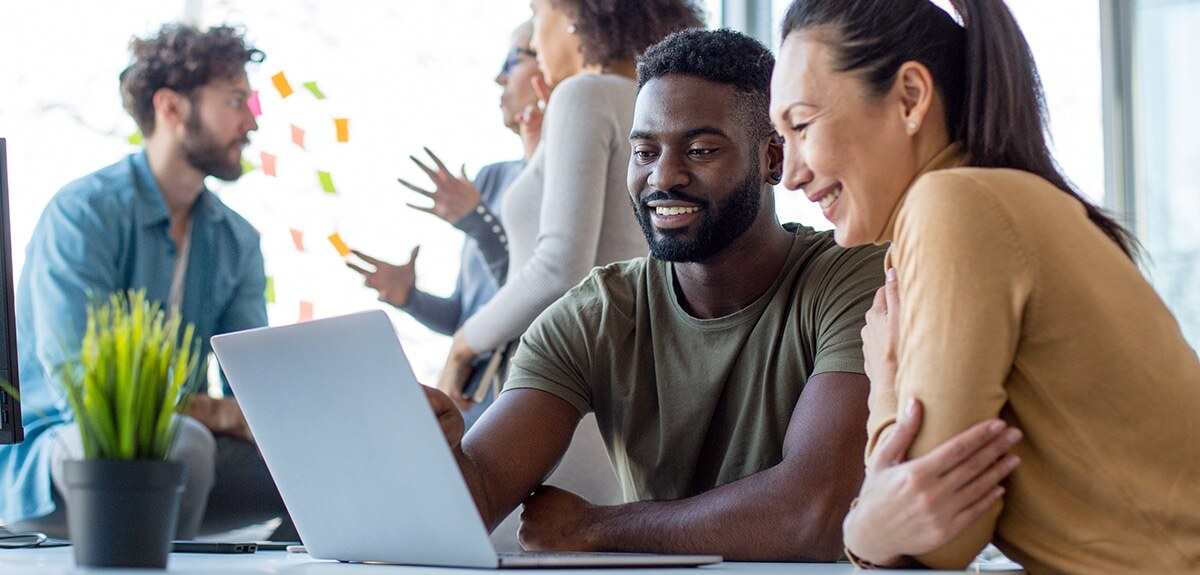
Customer feedback analysis: Using AI text analytics to turn comments into action
Are you getting the most out of your customer feedback data? AI-native text analytics helps brands make informed decisions and create better business outcomes.